2023国际计算智能最新进展会议受邀专家
专家 (按报告顺序排序) | 姓名 | 职称/职务 | 工作单位 | 报告标题 | 备注 |
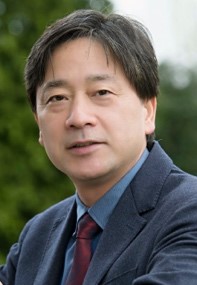
| 金耀初 | 欧洲科学院院士、IEEE会士、《Complex & Intelligent Systems》主编、IEEE 计算智能协会(CIS)候任主席、Web of Science(2019-2022)“全球高被引科学家”,教育部长江学者讲座教授 | 比勒费尔德大学(德国) | Graph Neural Networks for Combinatorial Optimization |
|
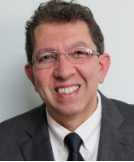
| Marios Polycarpou | 欧洲科学院院士、塞浦路斯科学、文学和艺术院院士、IEEE 会士、IFAC 会士,曾任IEEE计算智能协会(CIS)主席 (2012-2013)、《IEEE Transactions on Neural Networks and Learning Systems》主编 (2004-2010) | 塞浦路斯大学(塞浦路斯共和国) | Intelligent Fault Diagnostics in a Distributed World |
|
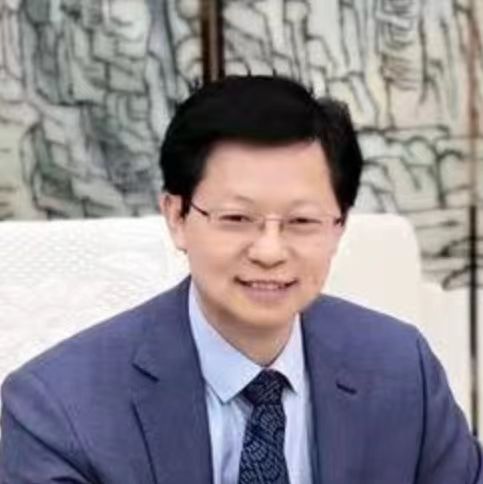
| 宋永端 | IEEE 会士、AAIA会士、国际欧亚科学院院士、中国自动化学会常务理事、重庆大学人工智能研究院院长,《IEEE Transactions on Neural Networks and Learning Systems》主编、《Journal of Automation and Intelligence》创刊主编 | 重庆大学 | Neural Networks Driven Control Design and Analysis | |
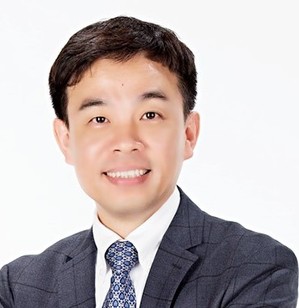
| Yiu-ming Cheung | IEEE 会士、AAAS 会士、IET 会士、British Computer Society (BCS) 研究员,被斯坦福大学评为人工智能和图像处理领域全球1%高被引用科学家(2019至今),长江学者讲座教授、《IEEE Transactions on Emerging Topics in Computational Intelligence》主编 | 香港浸会大学 | Imbalanced Data Learning: From Class Imbalance to Long-tailed Data Classification | |
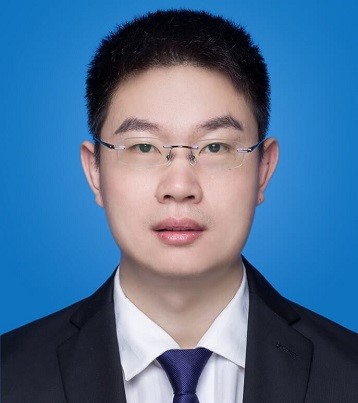
| 伍冬睿 | IEEE 会士、国家海外青年高层次人才,《IEEE Transactions on Fuzzy Systems》主编,曾任《IEEE Systems, Man, and Cybernetics Society》主编 (2021-2022)、爱思唯尔2021和2022高被引学者 | 华中科技大学 | Efficient Optimization of TSK Fuzzy System |
|
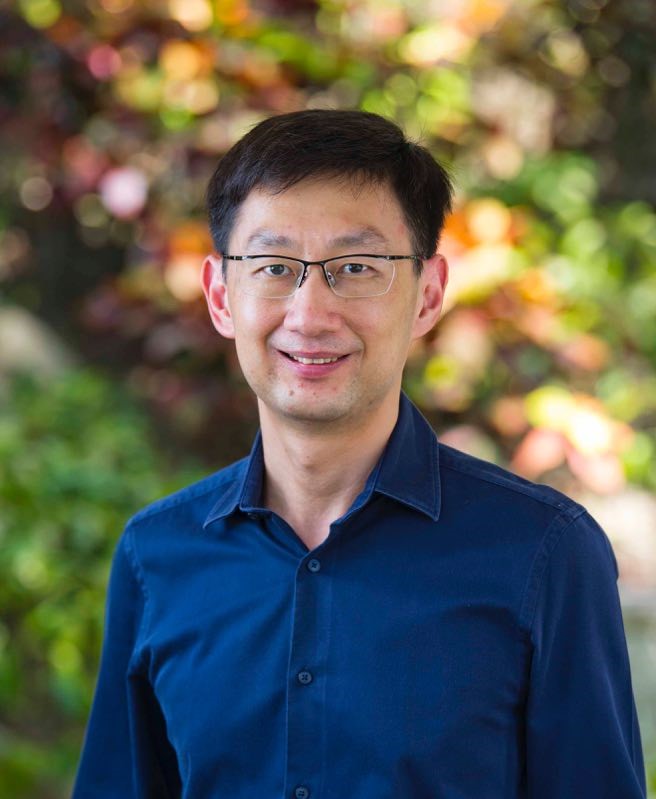
| Kay Chen Tan | IEEE计算智能协会(CIS)副主席、IEEE 会士、香港理工大学教授,斯普林格丛书《Machine Learning: Foundations, Methodologies, and Applications》联合主编,曾任《IEEE Transactions on Evolutionary Computation》主编 (2015-2020)、《IEEE Computational Intelligence Magazine》主编 (2010-2013) | 香港理工大学 | Towards Next-Generation Evolutionary Computation: Some Reflections |
|
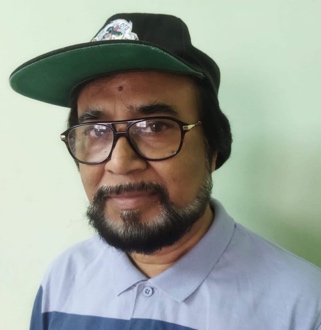 | Nikhil R. Pal | 印度国家工程院院士、印度国家科学院院士、发展中国家科学院院士、IEEE会士、国际模糊系统协会(IFSA)会士,曾任《IEEE Transactions on Fuzzy Systems》主编(2005-2010)、IEEE计算智能协会(CIS)主席(2018-2019) | 印度统计研究所(印度) | Neural Networks: Augmenting with Explainability, and Making Them Trustworthy and Bioinspired |
|
Graph Neural Networks for Combinatorial Optimization
Prof. Yaochu Jin
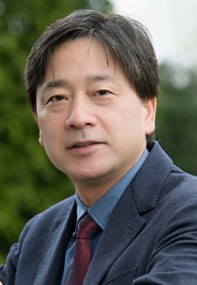
Member of Academia European, IEEE Fellow
Alexander von Humboldt Professor for Artificial Intelligence
Editor-in-Chief of Complex & Intelligent Systems
Bielefeld University, Germany
Abstract:Graph neural networks have been found successful in solving combinatorial optimization problems. This talk starts with a simple example of solving the travelling salesman problem using graph neural networks. Then, we present an approach to multi-objective facility location using two graph neural networks with supervised training. Finally, we showcase how a graph neural network with negative message passing can be trained using unsupervised training for solving graph coloring problems. We conclude the talk with a summary and discussion of future work.
Bio-Sketch: Yaochu Jin is an Alexander von Humboldt Professor for Artificial Intelligence endowed by the German Federal Ministry of Education and Research, with the Faculty of Technology, Bielefeld University, Germany. He is also a Distinguished Chair, Professor in Computational Intelligence, Department of Computer Science, University of Surrey, Guildford, U.K. He was a “Finland Distinguished Professor” of University of Jyväskylä, Finland, “Changjiang Distinguished Visiting Professor”, Northeastern University, China, and “Distinguished Visiting Scholar”, University of Technology Sydney, Australia. His main research interests include multi-objective and data-driven evolutionary optimization, evolutionary multi-objective learning, trustworthy AI, and evolutionary developmental AI.
Prof Jin is presently the President-Elect of the IEEE Computational Intelligence Society and the Editor-in-Chief of Complex & Intelligent Systems. He was named by the Web of Science as “a Highly Cited Researcher” from 2019 to 2022 consecutively. He is a Member of Academia Europaea and Fellow of IEEE.
Intelligent Fault Diagnostics in a Distributed World
Prof. Marios Polycarpou
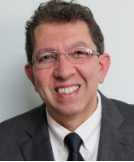
IEEE Fellow, IFAC Fellow, Academician, Founding Member of the Cyprus Academy of Sciences, Letters, and Arts, Academician,
Member of Academia Europaea (The Academy of Europe) Director, KIOS Research and Innovation Center of Excellence Professor,
Department of Electrical and Computer Engineering
University of Cyprus, Republic of Cyprus
Abstract: The emergence of interconnected cyber-physical systems and sensor/actuator networks has given rise to advanced automation applications, where a large amount of sensor data is collected and processed in order to make suitable real-time decisions and to achieve the desired control objectives. However, in situations where some components behave abnormally or become faulty, this may lead to serious degradation in performance or even to catastrophic system failures, especially due to cascaded effects of the interconnected subsystems. Distributed fault diagnosis refers to monitoring architectures where the overall system is viewed as an interconnection of various subsystems, each of which is monitored by a dedicated fault diagnosis agent that communicates and exchanges information with other “neighboring” agents. The goal of this presentation is to provide insight into various aspects of the design and analysis of intelligent monitoring and control schemes and to discuss directions for future research.
Bio-Sketch: Marios Polycarpou is a Professor of Electrical and Computer Engineering and the Director of the KIOS Research and Innovation Center of Excellence at the University of Cyprus. He is also a Founding Member of the Cyprus Academy of Sciences, Letters, and Arts, an Honorary Professor of Imperial College London, and a Member of Academia Europaea (The Academy of Europe). He received the B.A degree in Computer Science and the B.Sc. in Electrical Engineering, both from Rice University, USA in 1987, and the M.S. and Ph.D. degrees in Electrical Engineering from the University of Southern California, in 1989 and 1992 respectively. His teaching and research interests are in intelligent systems and networks, adaptive and learning control systems, fault diagnosis, machine learning, and critical infrastructure systems.
Prof. Polycarpou is the recipient of the 2023 IEEE Frank Rosenblatt Technical Field Award and the 2016 IEEE Neural Networks Pioneer Award. He is a Fellow of IEEE and IFAC. He served as the President of the IEEE Computational Intelligence Society (2012-2013), as the President of the European Control Association (2017-2019), and as the Editor-in-Chief of the IEEE Transactions on Neural Networks and Learning Systems (2004-2010). Prof. Polycarpou currently serves on the Editorial Boards of the Proceedings of the IEEE and the Annual Reviews in Control. His research work has been funded by several agencies and industry in Europe and the United States, including the prestigious European Research Council (ERC) Advanced Grant, the ERC Synergy Grant and the EU-Widening Teaming program.
Neural Networks Driven Control Design and Analysis
Prof. Yongduan Song
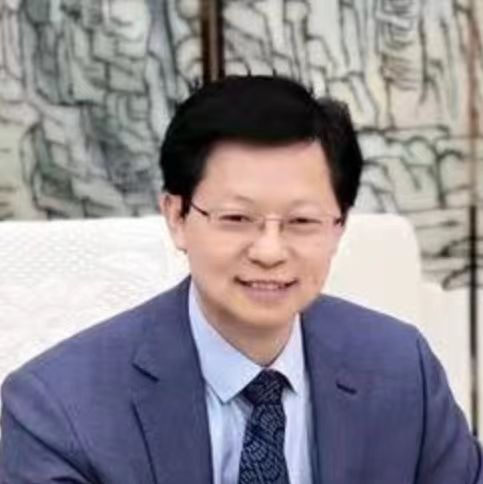
IEEE Fellow, Fellow of AAIA, Fellow of the International Eurasian Academy of Sciences, Registered Professional Engineer (USA), Fellow of Chinese Automation Association
Managing Director of the Chinese Academy of Automation
Chongqing University, Chongqing, China
Abstract: Neural networks and related learning algorithms are crucial components of artificial intelligence. The utilization of neural networks combined with learning algorithms for controller design has become a mainstream direction in the field of intelligent control. This talk will examine the typical NN driven design approaches and expose several critical issues related to functionality and effectiveness of the NN based control methods.
Bio-Sketch: Professor Yong-Duan Song is a Fellow of IEEE, Fellow of AAIA, Fellow of International Eurasian Academy of Sciences, and Fellow of Chinese Automation Association. He was one of the six Langley Distinguished Professors at National Institute of Aerospace (NIA), USA and register professional engineer (USA). He is currently the dean of Research Institute of Artificial Intelligence at Chongqing University. Professor Song is the Editor-in-Chief of IEEE Transactions on Neural Networks and Learning Systems (TNNLS) and the founding Editor-in-Chief of the International Journal of Automation and Intelligence.
Imbalanced Data Learning: From Class Imbalance to Long-tailed Data Classification
Prof. Yiu-ming Cheung
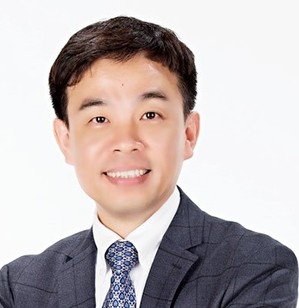
IEEE Fellow, AAAS Fellow, IET Fellow, and British Computer Society (BCS) Fellow, a Chair Professor (Artificial Intelligence) of the Department of Computer Science and Dean of Institute for Research and Continuing Education (IRACE) in Hong Kong Baptist University (HKBU)
the Founding Chairman of IEEE (Hong Kong) Computational Intelligence Chapter and the Chair of Technical Community on Intelligent Informatics (TCII) of IEEE Computer Society
Hong Kong Baptist University, Hong Kong
Abstract: In many practical problems, the number of data forming difference classes can be quite imbalanced. In particular, real-world data are often typically exhibiting a long-tailed distribution, which refers to a small number of classes with abundant training samples but the remaining large number of classes only with very few training instances. Such imbalanced data could make the performance of the most machine learning methods become deteriorate to a certain degree. In general, the problem of learning from imbalanced data is nontrivial and challenging in the field of data engineering and machine learning, which has attracted growing attentions in recent years. In this talk, we will introduce the imbalanced data learning and its related techniques, as well as its applications. In particular, our recent advances on this research area will introduced as well.
Bio-Sketch: Yiu-ming Cheung is currently a Chair Professor (Artificial Intelligence) of the Department of Computer Science and Dean of Institute for Research and Continuing Education (IRACE) in Hong Kong Baptist University (HKBU). He received PhD degree from Department of Computer Science and Engineering at The Chinese University of Hong Kong in 2000, and then joined the Department of Computer Science at HKBU in 2001. He is an IEEE Fellow, AAAS Fellow, IET Fellow, and British Computer Society (BCS) Fellow. He is the awardee of RGC Senior Research Fellow with receiving a fellowship grant of HK$7.8 million over a period of 60 months. Since 2019, he has been ranked the World’s Top 1% Most-cited Scientists in the field of Artificial Intelligence and Image Processing by Stanford University for consecutive five years. He was elected as a Distinguished Lecturer of IEEE Computational Intelligence Society in 2020, and named a Chair Professor of Changjiang Scholars Program by the Ministry of Education of the People’s Republic of China for the dedication and exceptional achievements in his academic career. Also, he is currently the Editor-in-Chief of IEEE Transactions on Emerging Topics in Computational Intelligence.
His research interests include machine learning and visual computing, as well as their applications in data science, pattern recognition, multi-objective optimization, and information security. He has published over 250 articles in the high-quality conferences and journals, including TPAMI, TNNLS, TIFS, TIP, TMM, TKDE, TCYB, CVPR, IJCAI, AAAI, and so on. His three co-authored papers have been selected as ESI Highly Cited Papers (i.e. listed in Top 1% globally in the corresponding discipline). Moreover, he has been granted one Chinese patent and two US patents. Subsequently, the underlying technique of his eye-gaze tracking patent has been successfully applied to develop the first mobile app for fatigue driving detection. It turns out that, selected from 1000 new inventions and products of 700+ competition teams from 40 countries, he was awarded two most prestigious prizes: (1) the Gold Medal with Distinction (i.e. the highest grade in Gold Medals) and (2) Swiss Automobile Club Prize, in the 45th International Exhibition of Invention, Geneva, Switzerland, on March 29-April 2, 2017, in recognition of his innovative work. Also, he was the Gold Award Winner of Hong Kong Innovative Invention Award in the Seventh Hong Kong Innovative Technologies Achievement Award 2017. In addition, he won the Gold Medal with Congratulations of Jury (i.e. the highest grade in Gold Medals) and the Award of Excellence from Romania, respectively, at the 46th International Exhibition of Inventions of Geneva 2018 with his invention “Lip-password: Double Security System for Identity Authentication”. He was the recipient of: (1) Best Research Award of Department of Computer Science at HKBU in 2011 and 2021, respectively, (2) Best in Theoretical Paper Award in WI-IAT’2020, (3) Best Paper Awards in SEAL’2017, ISICA’2017, ICNC-FSKD’2014, and IEEE IWDVT’2005, respectively, (4) Best Student Paper Award in ISMIS’2018, (5) 2017 IETI Annual Scientific Award, and (6) 2017-2019 Albert Nelson Marquis Lifetime Achievement Award.
He is the Founding Chairman of IEEE (Hong Kong) Computational Intelligence Chapter and the Chair of Technical Community on Intelligent Informatics (TCII) of IEEE Computer Society. He has served in various capacities (e.g., Organizing Committee Chair, Program Committee Chair, Program Committee Area Chair, and Financial Chair) at several top-tier international conferences, including IJCAI’2021, ICPR’2020, ICDM’2017 & 2018, WCCI’2016, WI-IAT’2012, ICDM’2006 & WI-IAT’2006, to name a few. He is an Associate Editor of several prestigious journals, including IEEE Transactions on Cybernetics, IEEE Transactions on Emerging Topics in Computational Intelligence, IEEE Transactions on Cognitive and Developmental Systems, IEEE Transactions on Neural Networks and Learning Systems (2014-2020), Pattern Recognition, Pattern Recognition Letters, Knowledge and Information Systems (KAIS), and Neurocomputing, as well as the Guest Editor in several international journals. Currently, he is an Engineering Panel member of Research Grants Council, Hong Kong, a member of assessment panel of Enterprise Support Scheme (ESS) under the Innovation and Technology Fund (ITF), and a Fellow Committee member of IEEE Computational Intelligence Society.
Efficient Optimization of TSK Fuzzy Systems
Prof. Dongrui Wu
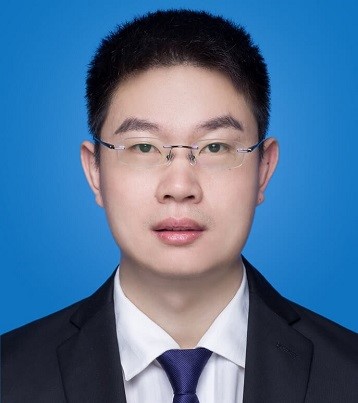
IEEE Fellow, the Editor-in-Chief of IEEE Transactions on Fuzzy Systems,
Professor and Deputy Director of the Key Laboratory of the Ministry of Education for Image Processing and Intelligent Control, School of Artificial Intelligence and Automation
Huazhong University of Science and Technology, Wuhan, China
Abstract: TSK fuzzy systems have been widely used in classification and regression. However, when the training set is very large, traditional evolutionary algorithm based optimization and gradient descent based optimization may have prohibitive computational cost. This talk first introduces the functional equivalence/similarity between TSK fuzzy systems and some classical machine learning models, including radial basis function networks, mixture of experts, stacking and CART, and then extends efficient training algorithms for deep neural networks, e.g., mini-batch gradient descent, DropOut, batch normalization, Adam, etc., to the training of TSK fuzzy systems, for better generalization.
Bio-Sketch: Dongrui Wu (IEEE Fellow) received a B.E in Automatic Control from the University of Science and Technology of China, Hefei, China, in 2003, an M.Eng in Electrical and Computer Engineering from the National University of Singapore in 2006, and a PhD in Electrical Engineering from the University of Southern California, Los Angeles, CA, in 2009. He is now Professor and Deputy Director of the Key Laboratory of the Ministry of Education for Image Processing and Intelligent Control, School of Artificial Intelligence and Automation, Huazhong University of Science and Technology, Wuhan, China.
Prof. Wu's research interests include brain-computer interface, machine learning, computational intelligence, and affective computing. He has more than 200 publications (11000+ Google Scholar citations; h=57). He received the IEEE Computational Intelligence Society (CIS) Outstanding PhD Dissertation Award in 2012, the IEEE Transactions on Fuzzy Systems Outstanding Paper Award in 2014, the IEEE Systems, Man and Cybernetics (SMC) Society Early Career Award in 2017, the USERN Prize in Formal Sciences in 2020, the IEEE Transactions on Neural Systems and Rehabilitation Engineering Best Paper Award in 2021, the Chinese Association of Automation Early Career Award in 2021, and the Ministry of Education Young Scientist Award in 2022. His team won National Champion of the China Brain-Computer Interface Competition in two successive years (2021-2022). Prof. Wu is the Editor-in-Chief of IEEE Transactions on Fuzzy Systems.
Towards Next-Generation Evolutionary Computation: Some Reflections
Prof. Kay Chen Tan
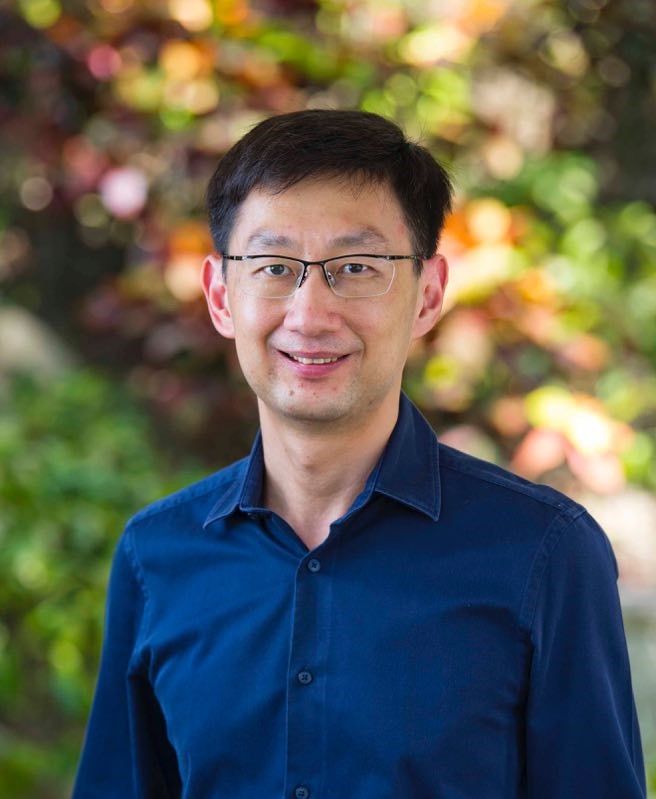
IEEE Fellow, Chair Professor (Computational Intelligence) of the Department of Computing, The Hong Kong Polytechnic University, Vice-President (Publications) of IEEE Computational Intelligence Society, Honorary Professor at University of Nottingham in UK, Chief Co-Editor of Springer Book Series on Machine Learning: Foundations, Methodologies, and Applications
Hong Kong Polytechnic University, Hong Kong, China
Abstract: Evolutionary computation refers to a set of stochastic search methods inspired by the principles of natural selection and genetics. It has demonstrated strong search capability in various applications, ranging from engineering design to financial forecasting and beyond. However, despite their success in some domains, there are several limitations when it comes to solving real-world search problems with high dimensionality, such as computational complexity, lack of robustness, and limited scalability.
It is known that deep machine learning has achieved remarkable breakthroughs in recent years, largely driven by rapid advancements in computing resources, the availability of big data, and the development of advanced algorithms. By learning from the success of deep machine learning, it is believed that the next generation of evolutionary algorithms should also leverage the availability of high-performance computing resources and other technologies to address the challenges of real-world problems. These algorithms should be more flexible, adaptive, and efficient, allowing them to provide optimal search solutions in a shorter amount of time.
In this talk, he will provide an overview of optimization in practical applications and and delve into the reasons behind developing the next generation of evolutionary algorithms. Specifically, he will introduce our research on evolutionary transfer optimization, where we focus on creating distributed, scalable, and learnable evolutionary algorithms to tackle challenging optimization problems. Furthermore, he will present an open-source evolutionary optimization platform that leverages the computational power of advanced hardware, such as GPU clusters, to enable users to develop high-performance evolutionary algorithms for solving real-world problems. Lastly, he will wrap up by discussing potential future research directions in this exciting field.
Bio-Sketch: Kay Chen Tan is currently a Chair Professor (Computational Intelligence) of the Department of Computing, The Hong Kong Polytechnic University. He has co-authored 9 books and published over 300 peer-reviewed journal articles. Prof. Tan serves as the Vice-President (Publications) of IEEE Computational Intelligence Society, USA from 2021-2024. He was the Editor-in-Chief of IEEE Transactions on Evolutionary Computation from 2015-2020 and IEEE Computational Intelligence Magazine from 2010-2013. Prof. Tan is an IEEE Fellow and an Honorary Professor at University of Nottingham in UK. He also serves as the Chief Co-Editor of Springer Book Series on Machine Learning: Foundations, Methodologies, and Applications.
Neural Networks: Augmenting with Explainability, and Making Them Trustworthy and Bioinspired
Prof. Nikhil R Pal
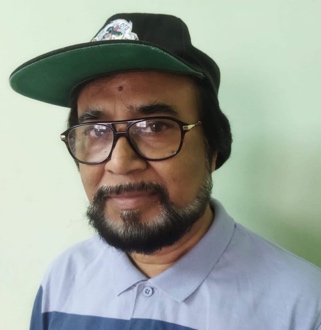
Centre for Artificial Intelligence and Machine Learning
Electronics and Communication Sciences Unit
Indian Statistical Institute
Calcutta, India
Abstract: This is an era of artificial intelligence (AI) and there are many success stories of AI, often beating human performance. In many cases, neural networks, in particular, deep neural networks, are the main pillars of such systems. But are these systems comprehensible and/or biologically plausible? In most cases, they are not! In my view, comprehensibility of a system depends, at least, on the following: simplicity, interpretability, explainability, trustworthiness, fairness, and biological plausibility of such systems, if that makes sense. Ideally, we should strive for realizing all these attributes in any intelligent system, but this is very difficult. So, we shall adopt an easier path to describe how some of these attributes may be realized separately. Particularly, we explain how a convolutional neural network can be augmented with explainability, how some observations in the visual cortex of a cat can be exploited to make a multi-layered perceptron a little more comprehensible. Finally, to make an MLP trustworthy, we shall discuss, how it can be equipped to say “Don’t know” in appropriate situations.
Bio-Sketch: Nikhil R. Pal is a Professor in the Electronics and Communication Sciences Unit and is associated with the Center for Artificial Intelligence and Machine Learning of the Indian Statistical Institute. His current research interest includes brain science, computational intelligence, machine learning, and data mining. He was the Editor-in-Chief of the IEEE Transactions on Fuzzy Systems for the period January 2005-December 2010. He served/has been serving on the editorial /advisory board/ steering committee of several journals including the International Journal of Approximate Reasoning, Applied Soft Computing, International Journal of Neural Systems, Fuzzy Sets and Systems, IEEE Transactions on Fuzzy Systems and the IEEE Transactions on Cybernetics.
He is a recipient of the 2015 IEEE Computational Intelligence Society (CIS) Fuzzy Systems Pioneer Award, He has given many plenary/keynote speeches in different premier international conferences in the area of computational intelligence. He has served as the General Chair, Program Chair, and co-Program chair of several conferences. He has been serving as a Distinguished Lecturer of the IEEE CIS (2010-2012, 2016-2018.2022-2024) and was a member of the Administrative Committee of the IEEE CIS (2010-2012). He served as the Vice-President for Publications of the IEEE CIS (2013-2016) and the President of the IEEE CIS (2018-2019).
He is a Fellow of the National Academy of Sciences, India, Indian National Academy of Engineering, Indian National Science Academy, International Fuzzy Systems Association (IFSA), The World Academy of Sciences, and a Fellow of the IEEE, USA.